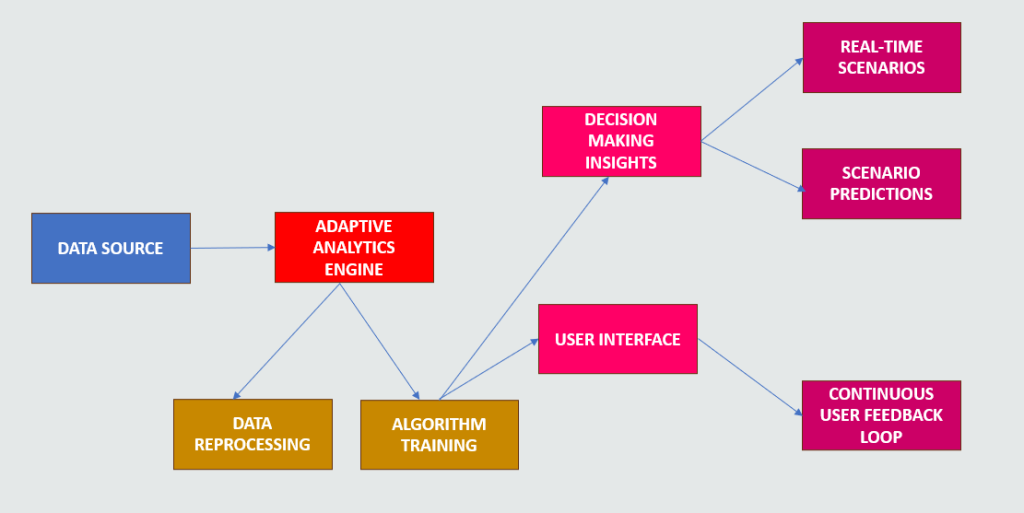
In today’s rapidly evolving digital landscape, organisations face challenges of making critical decisions based on incomplete, unstructured, and ever-changing data. Traditional predictive models often require extensive manual calibration, making them inefficient for dynamic environments. AI-Driven Adaptive Analytics presents an innovative solution by leveraging real-time predictive and prescriptive analytics, enabling businesses to make informed decisions with minimal human intervention. Predictive analytics systems currently in use provide valuable forecasts based on historical data but fail to offer actionable insights in real time. This limitation is particularly problematic in industries like supply chain, energy, and automation, where decision-making often relies on dynamic, real-time information. Existing solutions are typically static and require manual intervention to adjust models. This inflexibility makes them inefficient for addressing evolving, real-world conditions. While predictive models can indicate what might happen, they fall short in recommending how to act in response to changing scenarios. Our adaptive framework addresses these challenges by offering both predictive and prescriptive insights that evolve with new data inputs. This approach ensures that decision-makers have access to accurate, actionable recommendations, even in rapidly changing situations.
The Core Innovation: Responsive AI Algorithms
Here at FDMS Collective, a UK-based transformation start-up, we’ve initiated a project’s primary innovation focusing in the development of a responsive algorithm that can analyse and interpret dynamic data streams. Unlike conventional models that rely on structured datasets, this adaptive system can handle uncertainty and adjust its recommendations based on evolving data. By incorporating a layered, modular architecture, the AI system ensures flexibility and scalability, making it suitable for businesses across multiple industries. Our project proposes the development of a responsive algorithm that addresses a critical need in industries like supply chain, energy, and automation, where decision-making often needs to be based on incomplete or rapidly changing data. Current decision-making models rely on structured datasets and require manual intervention to maintain accuracy, making them unsuitable for fast-paced, data-driven environments. Our responsive algorithm continuously adapts to evolving data inputs, providing both predictive and prescriptive analytics that deliver real-time actionable insights.
Innovation and Differentiation
The specific innovation we propose is a self-correcting, adaptive system that integrates predictive and prescriptive analytics in a dynamic, real-time environment. Unlike traditional systems that struggle with unstructured or sparse data, our solution can handle incomplete datasets without requiring manual updates. The algorithm learns from real-time data, making automatic adjustments to provide recommendations aligned with the latest available information. This approach is game-changing because it not only predicts future outcomes but also prescribes the optimal actions based on the most current data, enabling industries to make data-driven decisions without delays or human oversight. The key difference between our solution and existing alternatives is in its adaptive nature. Traditional predictive models are static and require constant updates, limiting their ability to perform in dynamic environments. Our system, on the other hand, evolves with the data it receives, ensuring the decision-making process is always up-to-date and accurate. While other models forecast future events, they do not provide the actionable insights needed to make decisions in real time. Our system closes this gap by providing real-time recommendations that evolve with the data, significantly improving decision-making speed and accuracy. The core need our innovation addresses is the inability of current systems to process dynamic, incomplete, and unstructured data and provide actionable recommendations in real time. Supply chain, energy, and automation industries need solutions that can adapt to new data quickly, such as fluctuating market conditions, supply disruptions, and varying energy demands. Our solution provides an adaptive framework for real-time decision-making, significantly improving accuracy, speed, and operational efficiency across sectors. Pilot tests have already demonstrated that our solution can enhance decision-making in these complex, data-driven environments.
Key References Supporting This Innovation: Orlin, J. B., et al. (2018) explores the limitations of traditional predictive analytics, emphasising the gap between forecasting and real-time decision-making. Bannikov, B. S. (2020) highlights why conventional AI predictive models often fail, stressing the need for adaptive components to enhance accuracy and relevance. Sutton, R. S., & Barto, A. G. (2018) discuss the future of AI, advocating for a transition from purely predictive analytics to prescriptive decision support.
Key Features and Benefits
The system processes live data inputs to generate actionable insights, eliminating the need for time-consuming manual interventions. Designed for broad applicability, the AI model can be deployed in various sectors, including supply chain, energy, and automation, optimising inventory, forecasting demand, enhancing grid efficiency, and improving predictive maintenance. By continuously learning from new data, the model enhances its predictive accuracy over time, reducing errors and improving reliability. Companies adopting this AI system can expect reduced operational costs by preventing inefficiencies and identifying optimal strategies faster.
Societal and Economic Impact
The implementation of AI-Driven Adaptive Analytics aligns with broader economic and environmental goals, including sustainability, operational efficiency, and digital transformation. By reducing waste and optimising energy consumption, businesses can enhance productivity while maintaining cost-effectiveness.
The system is set to undergo pilot testing in the supply chain, energy, and automation sectors, with a structured roadmap to achieve full-scale commercial deployment within 12 months. By focusing on seamless integration and user-friendly interfaces, the AI platform ensures accessibility across industries. A subscription-based SaaS model will drive its monetisation, allowing businesses to scale usage based on their operational needs. AI-Driven Adaptive Analytics represents a paradigm shift in decision-making technology, offering businesses an intelligent, flexible, and scalable solution to navigate the complexities of modern data landscapes. As industries embrace AI-powered analytics, this innovation is poised to redefine efficiency, precision, and strategic execution on a global scale.
Target Market Structure and Dynamics
The target market spans supply chain, energy, and automation sectors, which are increasingly adopting AI-driven solutions to address decision-making challenges in environments with incomplete and dynamic data. The market is expanding rapidly due to the growing need for faster, more accurate decision-making to optimise operations and reduce costs.
Supply Chain: AI adoption is surging, with businesses adopting predictive analytics to optimise inventory, forecast demand, and improve route planning. The AI market for supply chain solutions is projected to reach $19.9 billion by 2027.
Energy: The energy sector’s adoption of AI for predictive maintenance, grid optimisation, and energy consumption forecasting is growing. AI is seen as critical for improving grid efficiency and integrating renewable energy sources, leading to a high-growth opportunity.
Automation: Manufacturing and industrial automation are investing heavily in AI for predictive maintenance and production optimisation, creating a vast market for real-time, adaptive decision systems.
Distinctiveness and Competitive Advantage
Our innovation stands out because it combines predictive and prescriptive analytics in a responsive, adaptive algorithm. Unlike existing AI models that require manual updates and rely on structured datasets, our system adapts in real-time to incomplete and unstructured data, providing actionable recommendations automatically. This sets us apart from competitors that lack real-time adaptability.
Our AI-Driven Adaptive Analytics is a breakthrough ininnovation and represents a paradigm shift in decision-making technology, offering businesses an intelligent, flexible, and scalable solution to navigate the complexities of modern data landscapes. As industries embrace AI-powered analytics, this innovation is poised to redefine efficiency, precision, and strategic execution on a global scale.